Regularized least squares¶
In the least squares problem, we minimized 2-norm squared of the data misfit relative to a linear model. In contrast to the least squares formulation, many problem need to balance competing objectives. For example, consider the problem of finding \vx_0 \in \R^n from noisy linear measurements \vb = \mA\vx_0 + \vw_\vb and \vg = \mF\vx_0-\vw_\vg. Here \vw_\vb and \vw_\vg are noise vectors. In order to solve this problem, we need to find a \vx that makes
- \func{f}_1(\vx) = \|\mA\vx - \vb\|_2^2 small, and
- \func{f}_2(\vx) = \|\mB\vx - \vg\|_2^2 small.
Generally, we can make \func{f}_1(\vx) or \func{f}_2(\vx) small, but not both. The figure below shows this relationship between \func{f}_1(\vx) and \func{f}_2(\vx).
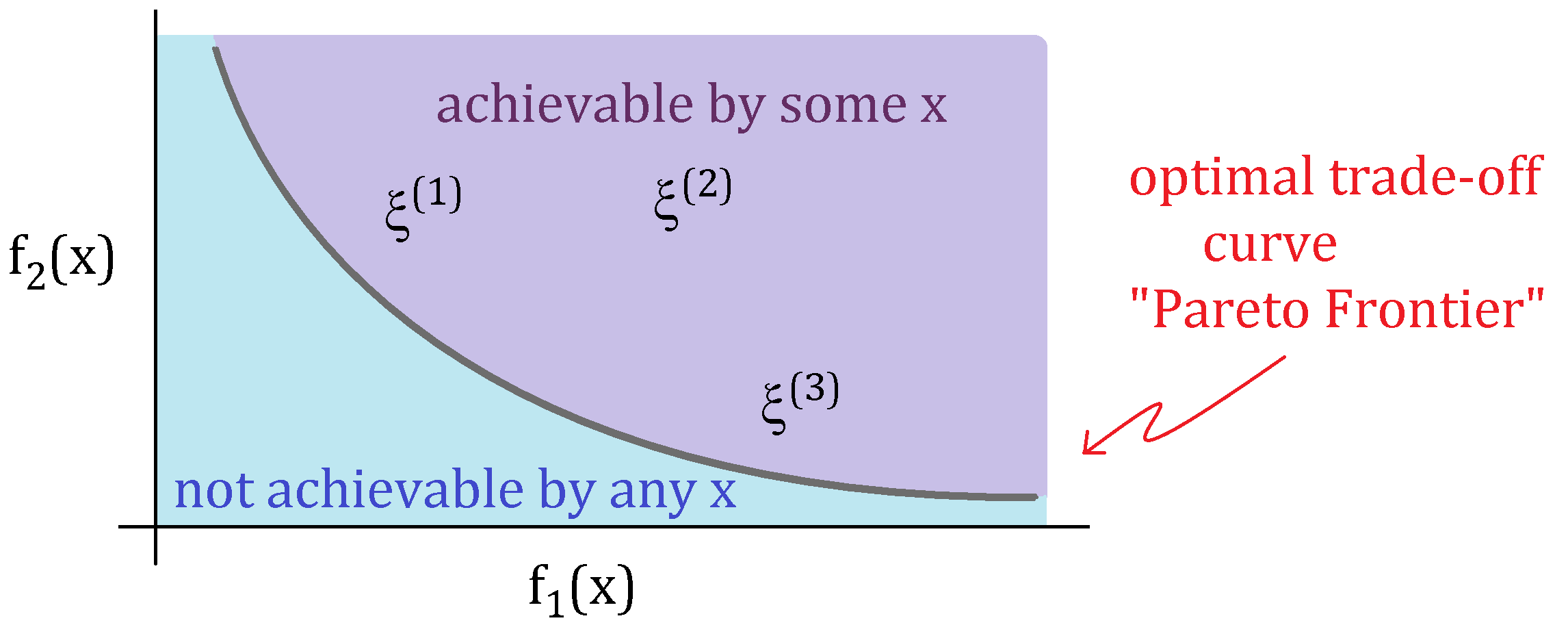
In the figure, the points in the boundary of two regions are called the Pareto optimal solutions. In order to find these optimal solutions, we minimize the following weighted sum objective:
The parameter \gamma is non-negative and defines relative weight between the objectives. For example, in the case of \gamma =1, the optimal point that minimizes \eqref{Regularized_LS_weight} is the point \vx on the optimal trade-off curve with f_1(\vx) = f_2(\vx).
Note that for a fixed \gamma and \alpha \in \R, the set
correspond to a line with slope of -\gamma. Another way to visualize the optimal solution is to find the line that is tangent to the optimal trade-off cuve, see figure below.
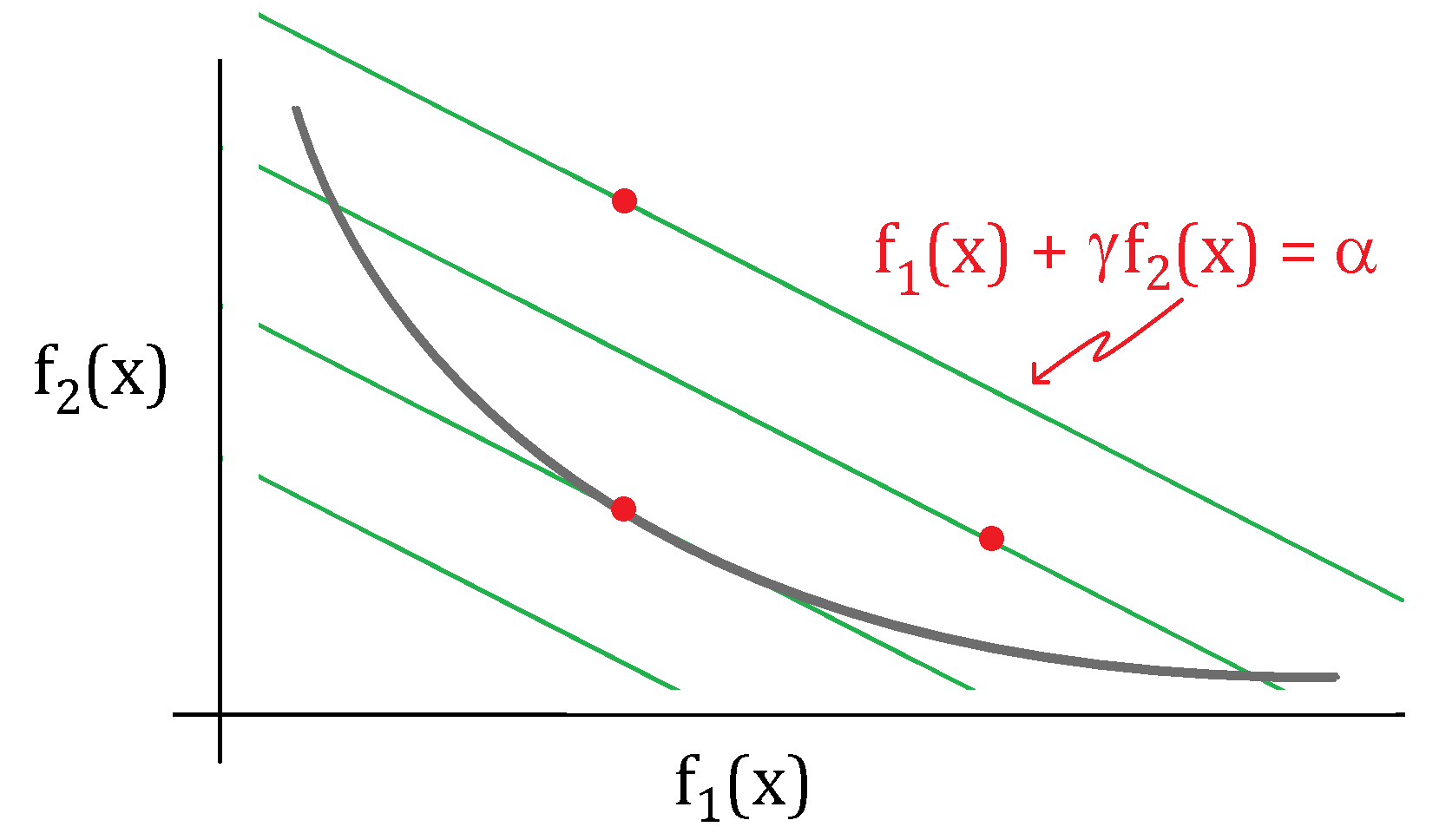
Example: Signal denoising¶
Suppose we observe noisy measurements of a signal:
where \hat{\vx}\in\R^n is the signal and \vw \in \R^n is noise. A simple apporach to find \hat{\vx} is to solve:
This minimization program doesnot enforce any structure on \vx. However, if we have prior information that the signal is "smooth", then we might balance the least squares fit against the smoothness of the solution in the following way:
Here, f_2(x) promotes smoothness. We can alternatively write the above minimization program in matrix notation. Define the finite differencem matrix
So, we can rewrite f_2(\vx) = \sum_{i=1}^{n-1}(x_i - x_{i+})^2 = \|\mD\vx\|_2^2. This allows for a reformulation of the weighted leas squares objective into a familiar least squares objective:
So the solution to the weighted least squares minimization program \eqref{Regularized_LS_identity} satisfies the normal equation \hat{\mA}\trans\hat{\mA}\vx = \hat{\mA}\trans\hat{\vb}, which simplifies to
Regularized least squares (aka Tikhonov)¶
We now generalize the result to noisy linear observations of a signal. In this case, the model is
where we added the measurement matrix \mA \in \R^{m\times n}. The corresponding wighted-sum least squares program is
where \|\mD\vx\|_2^2 is called the regularization penalty and \gamma is called the regularization parameter. The objective function can be reformulated as an least squares objective
and the corresponding normal equations is